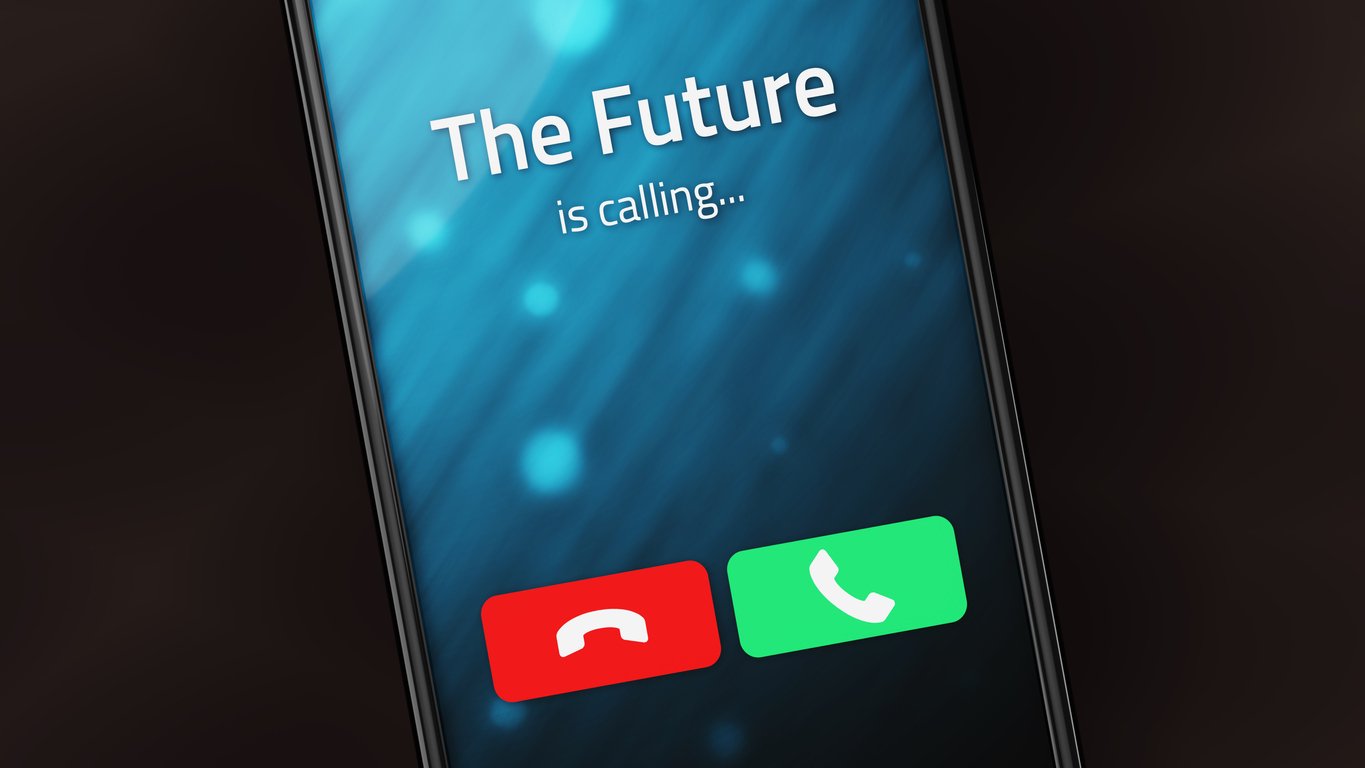
[Note from Nick]: Hey everybody! As a special treat, I’ve turned over the keys to the blog this week to our CTO, Andy Zimmerman. Enjoy!
I want you to imagine, for a moment, that it’s March 1986 and you are in a movie theater getting ready to watch Top Gun. During the previews, a time-traveling robot sits next to you, hands you some popcorn, and tells you to drop everything and invest in the upcoming Microsoft IPO. So, you run out of the theater, move your life savings into Microsoft stock (because who wouldn’t trust a time-traveling robot), and proceed to sit back and enjoy the ride.
Now, I want you to fast forward to August 2000. You’re having lunch with your very grateful stockbroker, and the time-traveling robot appears again. This time, it tells you to invest in this company called Enron… who was just named America’s Most Innovative Company for the sixth straight year. Given the roaring success of the robot’s last prediction, you whip out your new Nokia cell phone and sell hard on Microsoft in exchange for Enron.
16 months later, you are broke.
This is the inherent problem with time-traveling robots. When they tell the truth about the future, they can pave the way to stress-free living. But when they lie, they can lead to poor decision-making and catastrophic results.
In case you’re wondering, Nick did not invite me to be the first guest HumpDay Blogger in the last six years just to explain the inherent uncertainty of the stock market. But the story above mimics very closely the pain and distrust that many of our customers have felt when approaching the brave new world of Predictive Maintenance. While predictive machine learning and artificial intelligence algorithms promise to protect maintenance teams from costly downtime and expensive repairs by detecting impending equipment issues before failure occurs, many current technologies have fallen short because they provide seemingly bulletproof predictions that lack context, provide no room for error, and are often incorrect.
While Grace’s current suite of IIoT products are designed for real-time condition monitoring and to date have no truly predictive component (I’ll blame our marketing department for the “Predictive Maintenance” branding we use), over the past few years we have been working tightly with researchers at Iowa State University to develop a truly unique approach to predicting the failure of rolling element bearings in industrial applications. And I’m here today to shed just a little bit of light on that work!
Our research focus has been on building deep learning algorithms that can classify and predict bearing degradation while (a) leveraging physics-based insights to avoid the need for massive data sets, (b) providing probabilistic output that allows users to weigh contextual predictions with their own application knowledge, and (c) providing an explainable user interface that helps drive confidence by explaining how and why predictions are being made.
We believe that this work will lead to a world where maintenance personnel are told, for instance, that a bearing is 10% likely to fail in the next day, 50% likely to fail in the coming week, and 95% likely to fail in the coming month. This sort of information, especially when backed up with intuitive graphical explanations of why our model is predicting this specific failure, will give maintenance teams the ability to confidently use machine learning techniques to minimize risk without blindly following an algorithm they might not totally understand. Furthermore, our approach is lightweight enough to be deployed inside one of our wireless Vibration and Temperature Nodes. This means that in addition to performing hourly vibration analyses, our battery-powered nodes will provide hourly “remaining life” predictions without the need for expensive data processing equipment or extensive communications infrastructure.
While Grace is not quite ready to launch a true bearing prediction product just yet, some of our promising early results were just published in the journal Neurocomputing. You can get free access to this article through the end of February by using this link.
As our product line grows to include this sort of next-generation predictive capability, I hope to return to the HumpDay scene occasionally to share more exciting updates. In the meantime, feel free to reach out to our team to discuss how our existing product lines can help make your team safer, smarter, and more productive!
[Special thanks to my ISU colleagues Dr. Chao Hu, Dr. Venkat Nemani, and Mr. Hao Lu]
This material is based upon work supported by the National Science Foundation under STTR Grant Number 2036044 and PFI-TT Grant Number 1919265
NOW OPEN! Register today and submit YOUR questions to our panel of electrical safety experts. The next interactive Electrical Safety Panel Discussion will be presented by Terry Becker, Bhanu Srilla, Charles Miller, & John Kolak on Thursday, February 17.